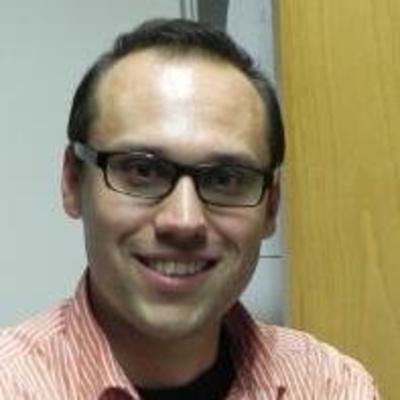
Dino Sejdinovic
Statistical machine learning, kernel methods, nonparametric statistics
I am an Associate Professor in Statistics at the University of Oxford, a Fellow of Mansfield College, and a Turing Fellow. I conduct research at the interface between machine learning and statistical methodology, with an emphasis on nonparametric and kernel methods.
Publications
2023
-
S. Bouabid
,
J. Fawkes
,
D. Sejdinovic
,
Returning The Favour: When Regression Benefits From Probabilistic Causal Knowledge, arXiv preprint arXiv:2301.11214, 2023.
2022
-
S. Bouabid
,
D. Watson-Parris
,
D. Sejdinovic
,
Bayesian inference for aerosol vertical profiles, in NeurIPS Workshop on Tackling Climate Change with Machine Learning, 2022.
-
S. Bouabid
,
D. Watson-Parris
,
S. Stefanović
,
A. Nenes
,
D. Sejdinovic
,
AODisaggregation: toward global aerosol vertical profiles, arXiv preprint arXiv:2205.04296, 2022.
-
J. Fawkes
,
R. J. Evans
,
D. Sejdinovic
,
Selection, ignorability and challenges with causal fairness, in Conference on Causal Learning and Reasoning, 2022, 275–289.
-
J. Fawkes
,
R. Evans
,
D. Sejdinovic
,
Selection, Ignorability and Challenges With Causal Fairness, arXiv preprint arXiv:2202.13774, 2022.
2021
-
S. L. Chau
,
S. Bouabid
,
D. Sejdinovic
,
Deconditional Downscaling with Gaussian Processes, in Advances in Neural Information Processing Systems (NeurIPS), 2021.
-
S. L. Chau
,
J. Ton
,
J. Gonzalez
,
Y. W. Teh
,
D. Sejdinovic
,
BayesIMP: Uncertainty Quantification for Causal Data Fusion, in Advances in Neural Information Processing Systems (NeurIPS), 2021.
-
R. Hu
,
G. K. Nicholls
,
D. Sejdinovic
,
Large Scale Tensor Regression using Kernels and Variational Inference, Machine Learning, 2021.
-
T. Fernandez
,
A. Gretton
,
D. Rindt
,
D. Sejdinovic
,
A Kernel Log-Rank Test of Independence for Right-Censored Data, Journal of the American Statistical Association, 2021.
-
V. Nguyen
,
S. B. Orbell
,
D. T. Lennon
,
H. Moon
,
F. Vigneau
,
L. C. Camenzind
,
L. Yu
,
D. M. Zumbühl
,
G. A. D. Briggs
,
M. A. Osborne
,
D. Sejdinovic
,
N. Ares
,
Deep Reinforcement Learning for Efficient Measurement of Quantum Devices, npj Quantum Information, vol. 7, no. 100, 2021.
-
A. Caterini
,
R. Cornish
,
D. Sejdinovic
,
A. Doucet
,
Variational Inference with Continuously-Indexed Normalizing Flows, in Uncertainty in Artificial Intelligence (UAI), 2021.
-
Z. Li
,
J. Ton
,
D. Oglic
,
D. Sejdinovic
,
Towards A Unified Analysis of Random Fourier Features, Journal of Machine Learning Research (JMLR), vol. 22, no. 108, 1–51, 2021.
-
X. Pu
,
S. L. Chau
,
X. Dong
,
D. Sejdinovic
,
Kernel-based Graph Learning from Smooth Signals: A Functional Viewpoint, IEEE Transactions on Signal and Information Processing over Networks, vol. 7, 192–207, 2021.
-
D. Rindt
,
D. Sejdinovic
,
D. Steinsaltz
,
Consistency of permutation tests of independence using distance covariance, HSIC and dHSIC, Stat, vol. 10, no. 1, e364, 2021.
-
J. Ton
,
L. Chan
,
Y. W. Teh
,
D. Sejdinovic
,
Noise Contrastive Meta Learning for Conditional Density Estimation using Kernel Mean Embeddings, in Artificial Intelligence and Statistics (AISTATS), 2021, PMLR 130:1099–1107.
-
J. Ton
,
D. Sejdinovic
,
K. Fukumizu
,
Meta Learning for Causal Direction, in Proceedings of the AAAI Conference on Artificial Intelligence, 2021, vol. 35, no. 11, 9897–9905.
-
R. Hu
,
D. Sejdinovic
,
Robust Deep Interpretable Features for Binary Image Classification, in Proceedings of the Northern Lights Deep Learning Workshop, 2021, vol. 2.
-
G. S. Blair
,
R. Bassett
,
L. Bastin
,
L. Beevers
,
M. I. Borrajo
,
M. Brown
,
S. L. Dance
,
A. Dionescu
,
L. Edwards
,
M. A. Ferrario
,
R. Fraser
,
H. Fraser
,
S. Gardner
,
P. Henrys
,
T. Hey
,
S. Homann
,
C. Huijbers
,
J. Hutchison
,
P. Jonathan
,
R. Lamb
,
S. Laurie
,
A. Leeson
,
D. Leslie
,
M. McMillan
,
V. Nundloll
,
O. Oyebamiji
,
J. Phillipson
,
V. Pope
,
R. Prudden
,
S. Reis
,
M. Salama
,
F. Samreen
,
D. Sejdinovic
,
W. Simm
,
R. Street
,
L. Thornton
,
R. Towe
,
J. V. Hey
,
M. Vieno
,
J. Waller
,
J. Watkins
,
The Role of Digital Technologies in Responding to the Grand Challenges of the Natural Environment: The Windermere Accord, Patterns, vol. 2, no. 1, 100156, 2021.
2020
-
D. Rindt
,
D. Sejdinovic
,
D. Steinsaltz
,
A kernel and optimal transport based test of independence between covariates and right-censored lifetimes, International Journal of Biostatistics, 2020.
-
N. M. Esbroeck
,
D. T. Lennon
,
H. Moon
,
V. Nguyen
,
F. Vigneau
,
L. C. Camenzind
,
L. Yu
,
D. Zumbuehl
,
G. A. D. Briggs
,
D. Sejdinovic
,
N. Ares
,
Quantum device fine-tuning using unsupervised embedding learning, New Journal of Physics, vol. 22, no. 9, 095003, 2020.
-
H. Moon
,
D. T. Lennon
,
J. Kirkpatrick
,
N. M. Esbroeck
,
L. C. Camenzind
,
L. Yu
,
F. Vigneau
,
D. M. Zumbühl
,
G. A. D. Briggs
,
M. A. Osborne
,
D. Sejdinovic
,
E. A. Laird
,
N. Ares
,
Machine learning enables completely automatic tuning of a quantum device faster than human experts, Nature Communications, vol. 11, no. 4161, 2020.
-
T. Rudner
,
D. Sejdinovic
,
Y. Gal
,
Inter-domain Deep Gaussian Processes, in International Conference on Machine Learning (ICML), 2020, PMLR 119:8286–8294.
-
D. Sejdinovic
,
Discussion of ‘Functional models for time-varying random objects’ by Dubey and Müller, Journal of the Royal Statistical Society: Series B, vol. 82, no. 2, 312–313, 2020.
2019
-
H. Law
,
P. Zhao
,
L. Chan
,
J. Huang
,
D. Sejdinovic
,
Hyperparameter Learning via Distributional Transfer, Advances in Neural Information Processing Systems (NeurIPS), to appear, 2019.
Project: tencent-lsml -
A. Raj
,
H. Law
,
D. Sejdinovic
,
M. Park
,
A Differentially Private Kernel Two-Sample Test, in European Conference on Machine Learning and Principles and Practice of Knowledge Discovery in Databases (ECML-PKDD), 2019, to appear.
Project: bigbayes -
D. Watson-Parris
,
S. Sutherland
,
M. Christensen
,
A. Caterini
,
D. Sejdinovic
,
P. Stier
,
Detecting Anthropogenic Cloud Perturbations with Deep Learning, in ICML 2019 Workshop on Climate Change: How Can AI Help?, 2019.
-
J. Runge
,
P. Nowack
,
M. Kretschmer
,
S. Flaxman
,
D. Sejdinovic
,
Detecting and Quantifying Causal Associations in Large Nonlinear Time Series Datasets, Science Advances, vol. 5, no. 11, 2019.
-
Z. Li
,
A. Perez-Suay
,
G. Camps-Valls
,
D. Sejdinovic
,
Kernel Dependence Regularizers and Gaussian Processes with Applications to Algorithmic Fairness, ArXiv e-prints:1911.04322, 2019.
-
D. Rindt
,
D. Sejdinovic
,
D. Steinsaltz
,
Nonparametric Independence Testing for Right-Censored Data using Optimal Transport, ArXiv e-prints:1906.03866, 2019.
-
J. Ton
,
L. Chan
,
Y. W. Teh
,
D. Sejdinovic
,
Noise Contrastive Meta-Learning for Conditional Density Estimation using Kernel Mean Embeddings, ArXiv e-prints:1906.02236, 2019.
Project: bigbayes tencent-lsml -
G. Camps-Valls
,
D. Sejdinovic
,
J. Runge
,
M. Reichstein
,
A Perspective on Gaussian Processes for Earth Observation, National Science Review, 2019.
-
Z. Li
,
J. Ton
,
D. Oglic
,
D. Sejdinovic
,
Towards A Unified Analysis of Random Fourier Features, in International Conference on Machine Learning (ICML), 2019, PMLR 97:3905–3914.
-
F. Briol
,
C. Oates
,
M. Girolami
,
M. Osborne
,
D. Sejdinovic
,
Probabilistic Integration: A Role in Statistical Computation? (with Discussion and Rejoinder), Statistical Science, vol. 34, no. 1, 1–22; rejoinder: 38–42, 2019.
2018
-
J. Mitrovic
,
D. Sejdinovic
,
Y. Teh
,
Causal Inference via Kernel Deviance Measures, in Advances in Neural Information Processing Systems (NeurIPS), 2018.
Project: bigbayes -
Q. Zhang
,
S. Filippi
,
A. Gretton
,
D. Sejdinovic
,
Large-Scale Kernel Methods for Independence Testing, Statistics and Computing, vol. 28, no. 1, 113–130, Jan. 2018.
Project: bigbayes -
A. Caterini
,
A. Doucet
,
D. Sejdinovic
,
Hamiltonian Variational Auto-Encoder, in Advances in Neural Information Processing Systems (NeurIPS), 2018, to appear.
-
H. Law
,
D. Sejdinovic
,
E. Cameron
,
T. Lucas
,
S. Flaxman
,
K. Battle
,
K. Fukumizu
,
Variational Learning on Aggregate Outputs with Gaussian Processes, in Advances in Neural Information Processing Systems (NeurIPS), 2018, to appear.
Project: bigbayes -
H. C. L. Law
,
P. Zhao
,
J. Huang
,
D. Sejdinovic
,
Hyperparameter Learning via Distributional Transfer, ArXiv e-prints:1810.06305, 2018.
Project: tencent-lsml -
M. Kanagawa
,
P. Hennig
,
D. Sejdinovic
,
B. Sriperumbudur
,
Gaussian Processes and Kernel Methods: A Review on Connections and Equivalences, ArXiv e-prints:1807.02582, 2018.
-
J. Ton
,
S. Flaxman
,
D. Sejdinovic
,
S. Bhatt
,
Spatial Mapping with Gaussian Processes and Nonstationary Fourier Features, Spatial Statistics, vol. 28, 59–78, 2018.
Project: bigbayes -
H. Law
,
D. Sutherland
,
D. Sejdinovic
,
S. Flaxman
,
Bayesian Approaches to Distribution Regression, in Artificial Intelligence and Statistics (AISTATS), 2018.
Project: bigbayes
2017
-
T. G. J. Rudner
,
D. Sejdinovic
,
Inter-domain Deep Gaussian Processes, NeurIPS 2017 Workshop on Bayesian Deep Learning, 2017.
-
S. Flaxman
,
Y. Teh
,
D. Sejdinovic
,
Poisson Intensity Estimation with Reproducing Kernels, Electronic Journal of Statistics, vol. 11, no. 2, 5081–5104, 2017.
Project: bigbayes -
Q. Zhang
,
S. Filippi
,
S. Flaxman
,
D. Sejdinovic
,
Feature-to-Feature Regression for a Two-Step Conditional Independence Test, in Uncertainty in Artificial Intelligence (UAI), 2017.
Project: bigbayes -
J. Mitrovic
,
D. Sejdinovic
,
Y. W. Teh
,
Deep Kernel Machines via the Kernel Reparametrization Trick, in International Conference on Learning Representations (ICLR) Workshop Track, 2017.
Project: bigbayes -
H. Law
,
C. Yau
,
D. Sejdinovic
,
Testing and Learning on Distributions with Symmetric Noise Invariance, in Advances in Neural Information Processing Systems (NeurIPS), 2017, 1343–1353.
-
J. Runge
,
P. Nowack
,
M. Kretschmer
,
S. Flaxman
,
D. Sejdinovic
,
Detecting Causal Associations in Large Nonlinear Time Series Datasets, ArXiv e-prints:1702.07007, 2017.
-
I. Schuster
,
H. Strathmann
,
B. Paige
,
D. Sejdinovic
,
Kernel Sequential Monte Carlo, in European Conference on Machine Learning and Principles and Practice of Knowledge Discovery in Databases (ECML-PKDD), 2017.
-
S. Flaxman
,
Y. W. Teh
,
D. Sejdinovic
,
Poisson Intensity Estimation with Reproducing Kernels, in Artificial Intelligence and Statistics (AISTATS), 2017.
Project: bigbayes
2016
-
D. Vukobratovic
,
D. Jakovetic
,
V. Skachek
,
D. Bajovic
,
D. Sejdinovic
,
G. Karabulut Kurt
,
C. Hollanti
,
I. Fischer
,
CONDENSE: A Reconfigurable Knowledge Acquisition Architecture for Future 5G IoT, IEEE Access, vol. 4, 3360–3378, 2016.
-
D. Vukobratovic
,
D. Jakovetic
,
V. Skachek
,
D. Bajovic
,
D. Sejdinovic
,
Network Function Computation as a Service in Future 5G Machine Type Communications, in International Symposium on Turbo Codes & Iterative Information Processing (ISTC), 2016, 365–369.
-
J. Mitrovic
,
D. Sejdinovic
,
Y. W. Teh
,
DR-ABC: Approximate Bayesian Computation with Kernel-Based Distribution Regression, in International Conference on Machine Learning (ICML), 2016, 1482–1491.
Project: bigbayes -
G. Franchi
,
J. Angulo
,
D. Sejdinovic
,
Hyperspectral Image Classification with Support Vector Machines on Kernel Distribution Embeddings, in IEEE International Conference on Image Processing (ICIP), 2016, 1898–1902.
-
B. Paige
,
D. Sejdinovic
,
F. Wood
,
Super-Sampling with a Reservoir, in Uncertainty in Artificial Intelligence (UAI), 2016, 567–576.
-
S. Flaxman
,
D. Sejdinovic
,
J. Cunningham
,
S. Filippi
,
Bayesian Learning of Kernel Embeddings, in Uncertainty in Artificial Intelligence (UAI), 2016, 182–191.
Project: bigbayes -
M. Park
,
W. Jitkrittum
,
D. Sejdinovic
,
K2-ABC: Approximate Bayesian Computation with Kernel Embeddings, in Artificial Intelligence and Statistics (AISTATS), 2016, 398–407.
2015
-
H. Strathmann
,
D. Sejdinovic
,
M. Girolami
,
Unbiased Bayes for Big Data: Paths of Partial Posteriors, ArXiv e-prints:1501.03326, 2015.
-
H. Strathmann
,
D. Sejdinovic
,
S. Livingstone
,
Z. Szabo
,
A. Gretton
,
Gradient-free Hamiltonian Monte Carlo with Efficient Kernel Exponential Families, in Advances in Neural Information Processing Systems (NeurIPS), vol. 28, 2015, 955–963.
-
K. Chwialkowski
,
A. Ramdas
,
D. Sejdinovic
,
A. Gretton
,
Fast Two-Sample Testing with Analytic Representations of Probability Measures, in Advances in Neural Information Processing Systems (NeurIPS), vol. 28, 2015, 1981–1989.
-
D. Vukobratovic
,
D. Sejdinovic
,
A. Pizurica
,
Compressed Sensing Using Sparse Binary Measurements: A Rateless Coding Perspective, in IEEE International Workshop on Signal Processing Advances in Wireless Communications (SPAWC), 2015.
-
Z. Kurth-Nelson
,
G. Barnes
,
D. Sejdinovic
,
R. Dolan
,
P. Dayan
,
Temporal structure in associative retrieval, eLife, vol. 4, no. e04919, 2015.
-
W. Jitkrittum
,
A. Gretton
,
N. Heess
,
S. M. A. Eslami
,
B. Lakshminarayanan
,
D. Sejdinovic
,
Z. Szabó
,
Kernel-Based Just-In-Time Learning for Passing Expectation Propagation Messages, in Uncertainty in Artificial Intelligence (UAI), 2015.
2014
-
K. Chwialkowski
,
D. Sejdinovic
,
A. Gretton
,
A Wild Bootstrap for Degenerate Kernel Tests, in Advances in Neural Information Processing Systems (NeurIPS), vol. 27, 2014, 3608–3616.
-
D. Sejdinovic
,
H. Strathmann
,
M. Lomeli
,
C. Andrieu
,
A. Gretton
,
Kernel Adaptive Metropolis-Hastings, in International Conference on Machine Learning (ICML), 2014, 1665–1673.
-
O. Johnson
,
D. Sejdinovic
,
J. Cruise
,
R. Piechocki
,
A. Ganesh
,
Non-Parametric Change-Point Estimation using String Matching Algorithms, Methodology and Computing in Applied Probability, vol. 16, no. 4, 987–1008, 2014.
2013
-
D. Sejdinovic
,
B. Sriperumbudur
,
A. Gretton
,
K. Fukumizu
,
Equivalence of distance-based and RKHS-based statistics in hypothesis testing, Annals of Statistics, vol. 41, no. 5, 2263–2291, Oct. 2013.
-
D. Sejdinovic
,
A. Gretton
,
W. Bergsma
,
A Kernel Test for Three-Variable Interactions, in Advances in Neural Information Processing Systems (NeurIPS), vol. 26, 2013, 1124–1132.
2012
-
A. Gretton
,
B. K. Sriperumbudur
,
D. Sejdinovic
,
H. Strathmann
,
S. Balakrishnan
,
M. Pontil
,
K. Fukumizu
,
Optimal Kernel Choice for Large-Scale Two-Sample Tests, in Advances in Neural Information Processing Systems (NeurIPS), vol. 25, 2012, 1205–1213.
-
D. Sejdinovic
,
A. Gretton
,
B. K. Sriperumbudur
,
K. Fukumizu
,
Hypothesis testing using pairwise distances and associated kernels, in International Conference on Machine Learning (ICML), 2012, 1111–1118.
-
R. Piechocki
,
D. Sejdinovic
,
Combinatorial Channel Signature Modulation for Wireless ad-hoc Networks, in IEEE International Conference on Communications (ICC), 2012.
-
A. Muller
,
D. Sejdinovic
,
R. Piechocki
,
Approximate Message Passing under Finite Alphabet Constraints, in IEEE International Conference on Acoustics, Speech, and Signal Processing (ICASSP), 2012.
2011
-
W. Dai
,
D. Sejdinovic
,
O. Milenkovic
,
Gaussian Dynamic Compressive Sensing, in International Conference on Sampling Theory and Applications (SampTA), 2011.
2010
-
D. Sejdinovic
,
O. Johnson
,
Note on noisy group testing: asymptotic bounds and belief propagation reconstruction, in 48th Annual Allerton Conference on Communication, Control, and Computing, 2010, 998–1003.
-
D. Sejdinovic
,
R. Piechocki
,
A. Doufexi
,
M. Ismail
,
Decentralised distributed fountain coding: asymptotic analysis and design, IEEE Communications Letters, vol. 14, no. 1, 42–44, 2010.
-
D. Sejdinovic
,
C. Andrieu
,
R. Piechocki
,
Bayesian sequential compressed sensing in sparse dynamical systems, in 48th Annual Allerton Conference on Communication, Control, and Computing, 2010, 1730–1736.
2009
-
D. Sejdinovic
,
D. Vukobratovic
,
A. Doufexi
,
V. Senk
,
R. Piechocki
,
Expanding window fountain codes for unequal error protection, IEEE Transactions on Communications, vol. 57, no. 9, 2510–2516, 2009.
-
D. Vukobratovic
,
V. Stankovic
,
D. Sejdinovic
,
L. Stankovic
,
Z. Xiong
,
Scalable video multicast using expanding window fountain codes, IEEE Transactions on Multimedia, vol. 11, no. 6, 1094–1104, 2009.
-
D. Sejdinovic
,
R. Piechocki
,
A. Doufexi
,
M. Ismail
,
Fountain code design for data multicast with side information, IEEE Transactions on Wireless Communications, vol. 8, no. 10, 5155–5165, 2009.
-
D. Sejdinovic
,
R. Piechocki
,
A. Doufexi
,
AND-OR tree analysis of distributed LT codes, in IEEE Information Theory Workshop (ITW), 2009, 261–265.
-
D. Vukobratovic
,
V. Stankovic
,
L. Stankovic
,
D. Sejdinovic
,
Precoded EWF codes for unequal error protection of scalable video, in International ICST Mobile Multimedia Communications Conference (MOBIMEDIA), 2009.
-
D. Sejdinovic
,
R. Piechocki
,
A. Doufexi
,
Rateless distributed source code design, in International ICST Mobile Multimedia Communications Conference (MOBIMEDIA), 2009.
-
D. Sejdinovic
,
Topics in Fountain Coding, PhD thesis, University of Bristol, 2009.
2008
-
D. Vukobratovic
,
V. Stankovic
,
D. Sejdinovic
,
L. Stankovic
,
Z. Xiong
,
Expanding window fountain codes for scalable video multicast, in IEEE International Conference on Multimedia and Expo (ICME), 2008, 77–80.
-
D. Sejdinovic
,
R. Piechocki
,
A. Doufexi
,
M. Ismail
,
Fountain coding with decoder side information, in IEEE International Conference on Communications (ICC), 2008, 4477–4482.
-
D. Sejdinovic
,
V. Ponnampalam
,
R. Piechocki
,
A. Doufexi
,
The throughput analysis of different IR-HARQ schemes based on fountain codes, in IEEE Wireless Communications and Networking Conference (WCNC), 2008, 267–272.
-
D. Sejdinovic
,
R. Piechocki
,
A. Doufexi
,
M. Ismail
,
Rate adaptive binary erasure quantization with dual fountain codes, in IEEE Global Telecommunications Conference (GLOBECOM), 2008.
2007
-
D. Vukobratovic
,
V. Stankovic
,
D. Sejdinovic
,
L. Stankovic
,
Z. Xiong
,
Scalable data multicast using expanding window fountain codes, in 45th Annual Allerton Conference on Communication, Control, and Computing, 2007.
-
D. Sejdinovic
,
D. Vukobratovic
,
A. Doufexi
,
V. Senk
,
R. Piechocki
,
Expanding window fountain codes for unequal error protection, in Asilomar Conference on Signals, Systems and Computers, 2007, 1020–1024.
Software
2017
-
S. Flaxman
,
Y. W. Teh
,
D. Sejdinovic
,
Kernel Poisson. 2017.
Project: bigbayes