We are a diverse group of researchers spanning many interests across machine learning, computational statistics and statistical methodology. There are ten faculty members spread over three overlapping subgroups.
Faculty
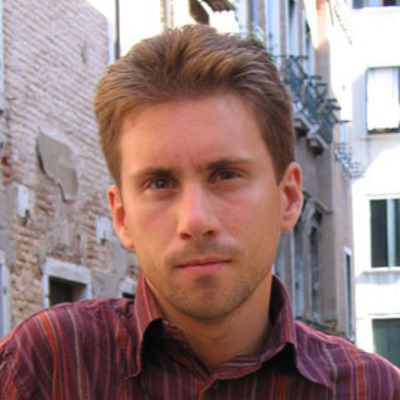
François Caron
Statistical Machine Learning, Bayesian methods, Bayesian nonparametrics, Statistical Network Analysis
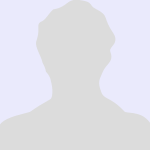
George Deligiannidis
Computational Statistics, Monte Carlo methods
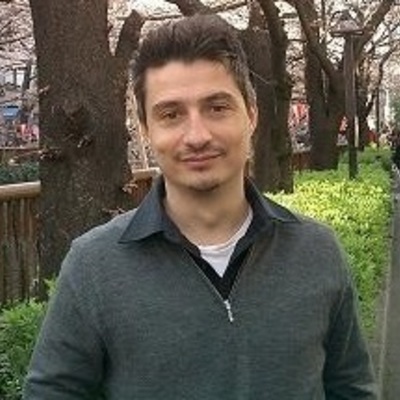
Arnaud Doucet
Computational Statistics, Monte Carlo methods
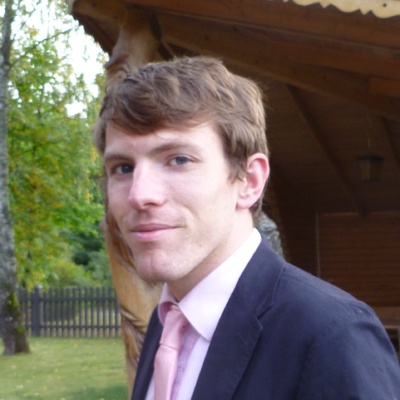
Robin Evans
Graphical models, causality, algebraic statistics
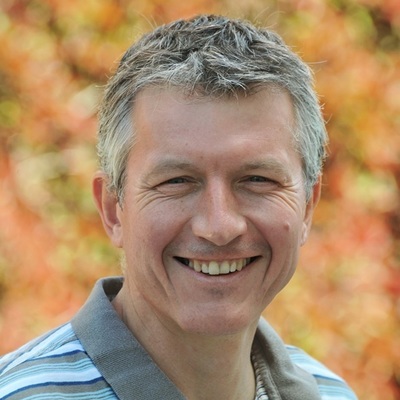
Chris Holmes
Decision theory, biostatistics and precision medicine, probabilistic learning under model misspecification
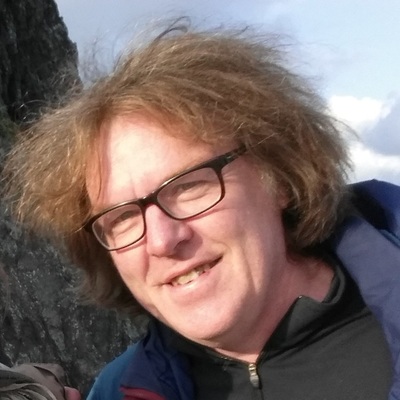
Geoff Nicholls
Statistical modeling, Bayes Methods, Monte Carlo Methods.
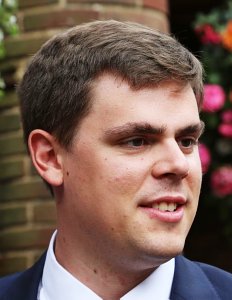
Tom Rainforth
Machine learning, Bayesian inference, Probabilistic programming, Deep generative models
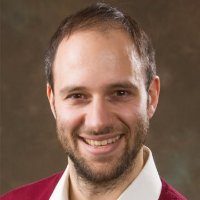
Patrick Rebeschini
Learning theory, Optimization, Implicit Regularization
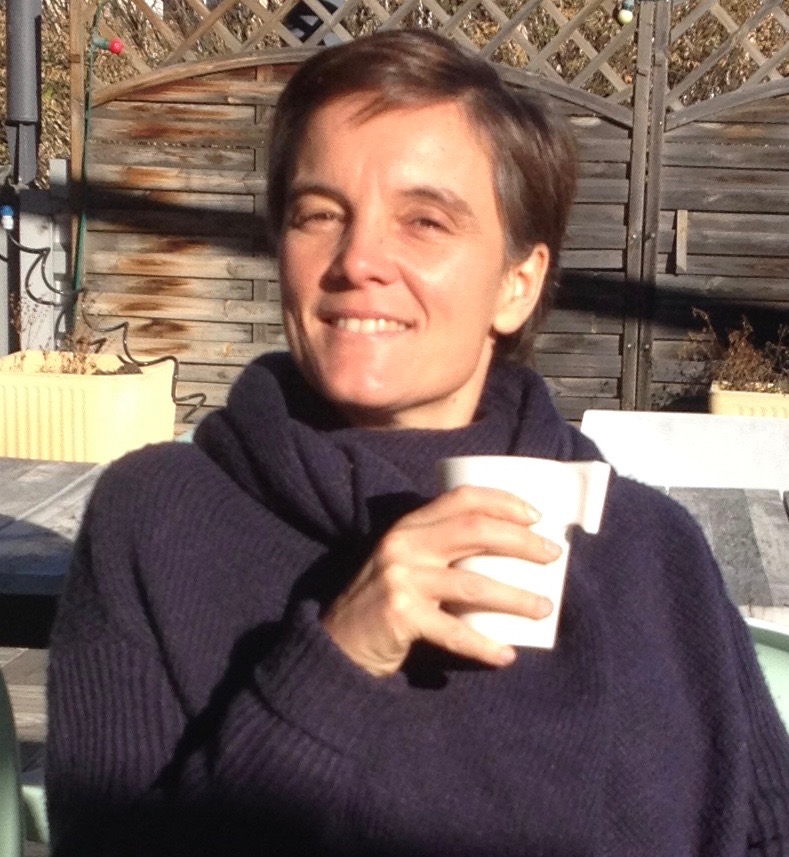
Judith Rousseau
Bayesian statistics, Asymptotics, Nonparametric statistics
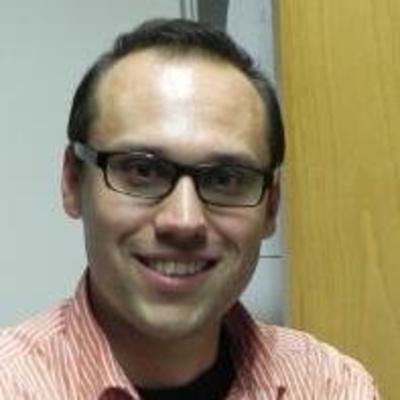
Dino Sejdinovic
Statistical machine learning, kernel methods, nonparametric statistics
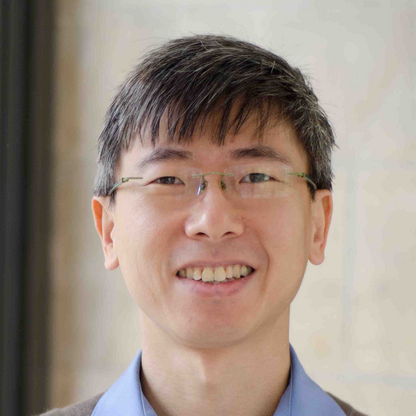
Yee Whye Teh
Bayesian nonparametrics, probabilistic learning, deep learning
Affiliated Faculty
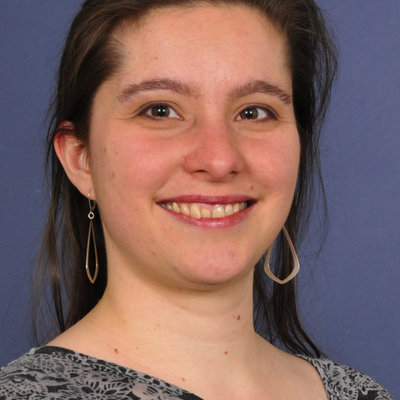
Sarah Filippi
Statistical machine learning and Bayesian statistics motivated by applications in biomedicine
Post-docs
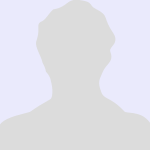
M. Azim Ansari
Statistical Genetics, Evolution, Host Pathogen Interactions, Computational Biostatistics, Machine Learning, Bayesian Statistics
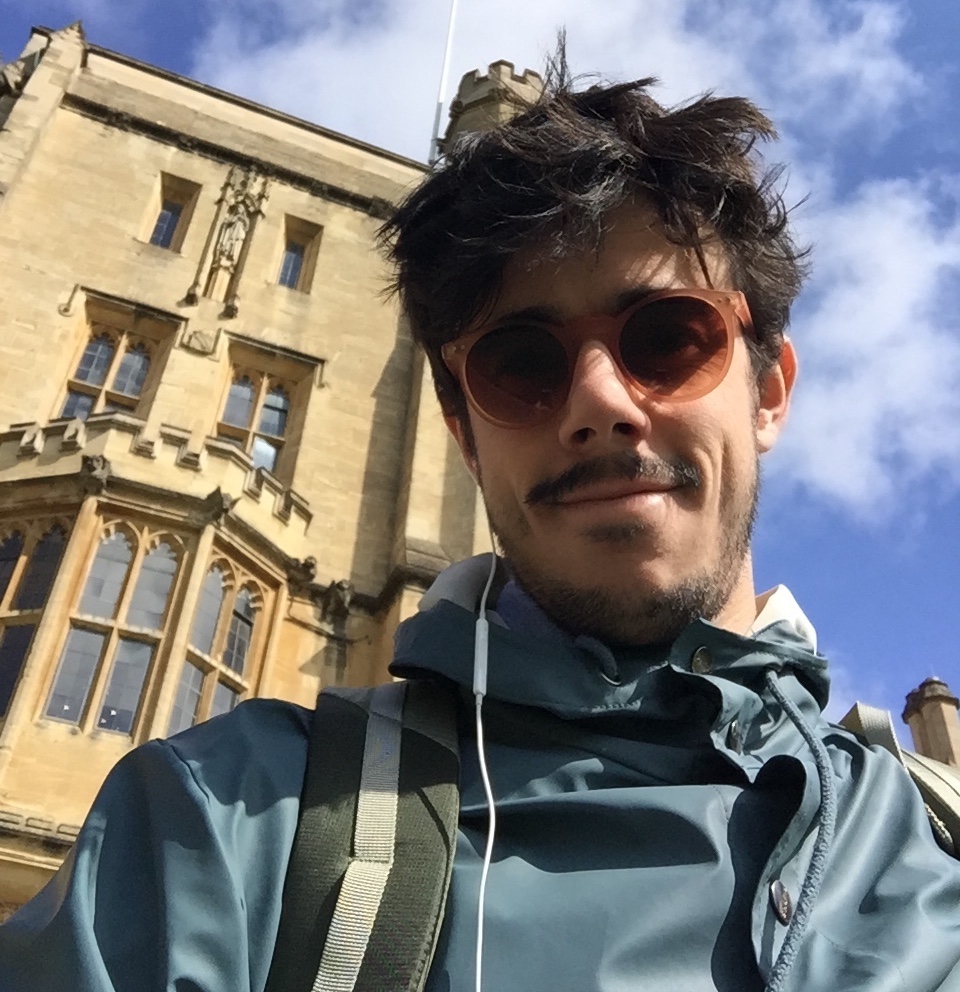
Emile Mathieu
Probabilistic inference, Deep learning, Generative models, Representation Learning, Geometry
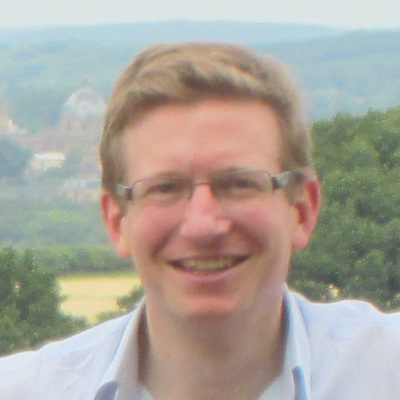
George Nicholson
Computational biostatistics, machine learning, precision medicine
Graduate Students
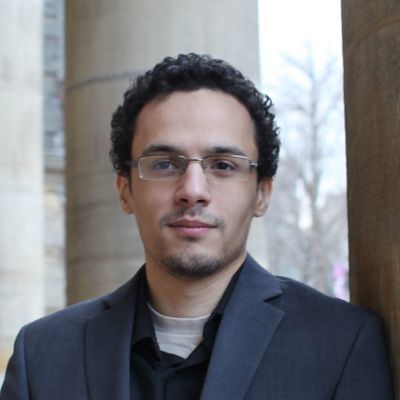
Moustafa Abdalla
Multi-view Learning, Time-series modelling, Statistical Genetics, Drug Development, High-throughput screening
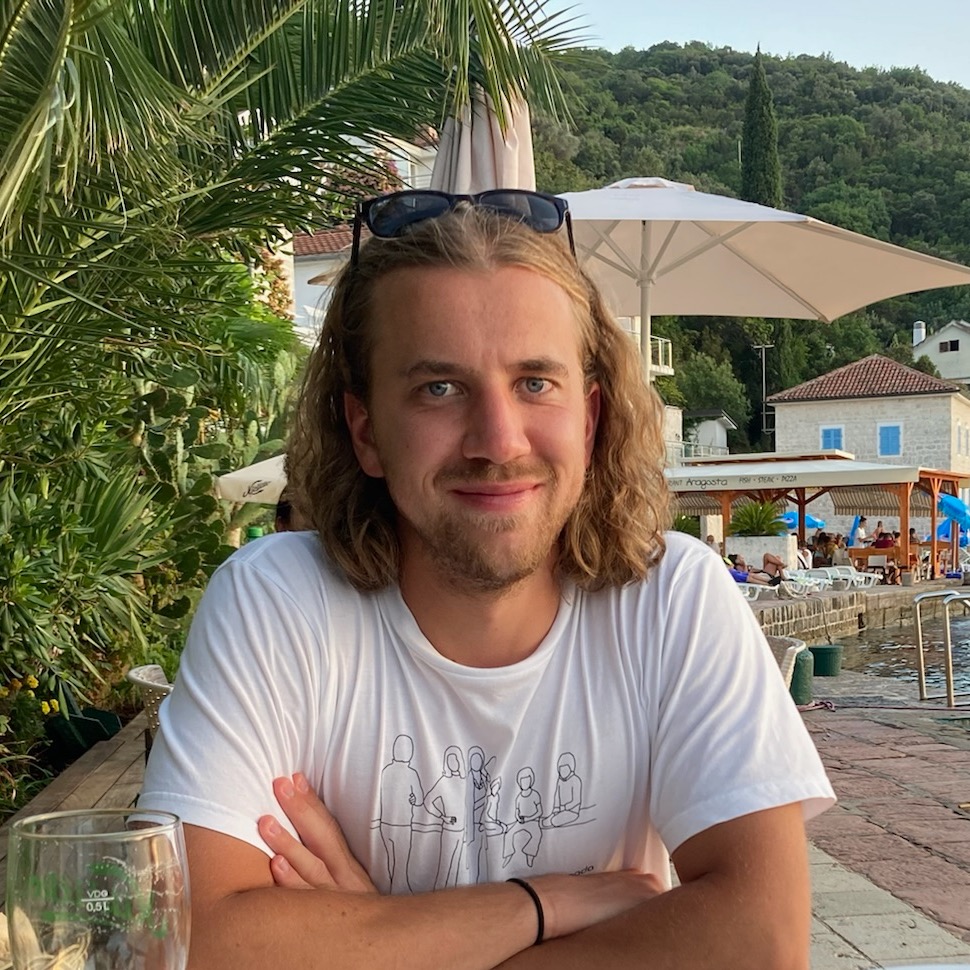
Freddie Bickford Smith
Deep Learning, Uncertainty Estimation
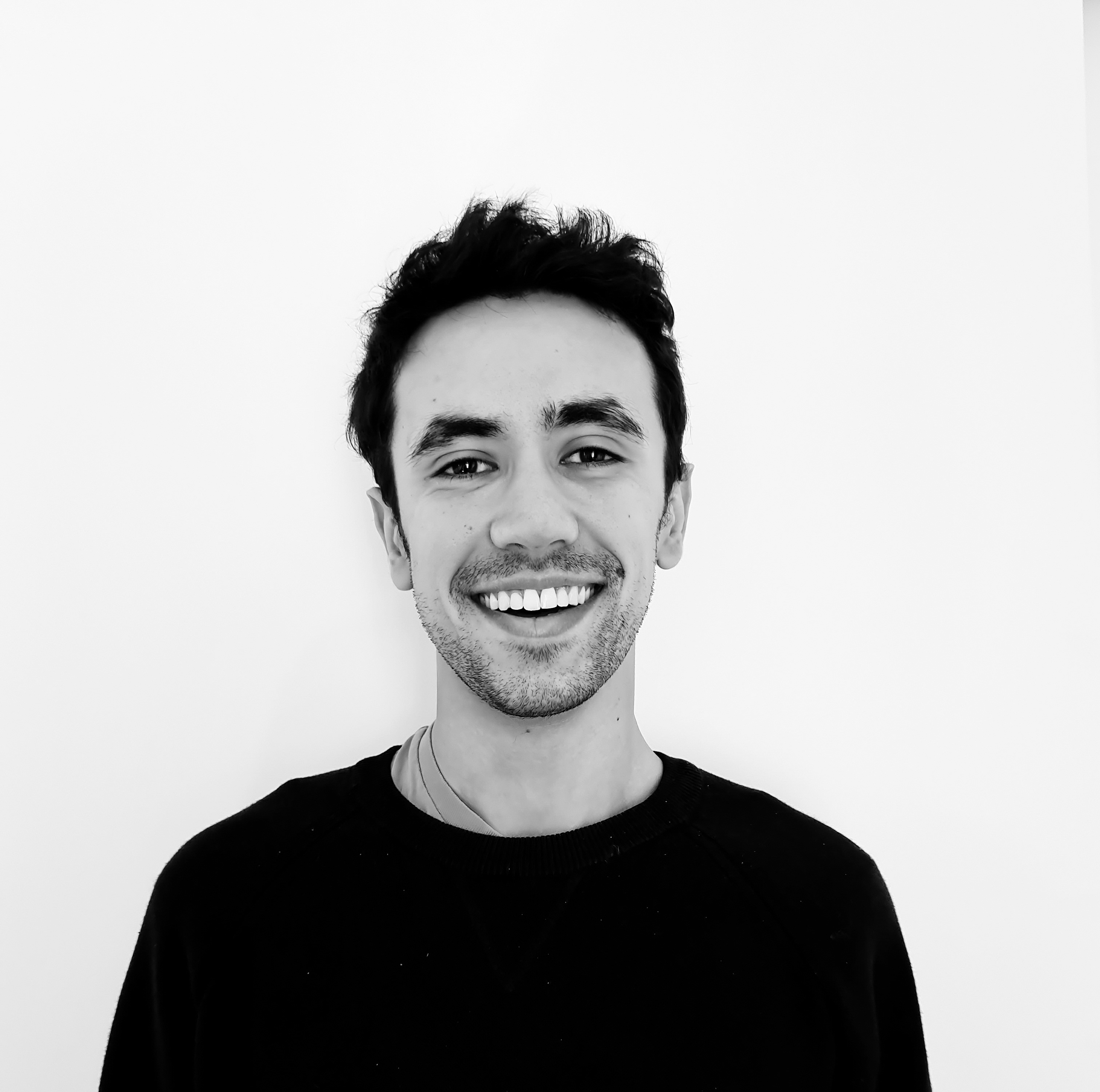
Shahine Bouabid
Kernel Methods, Gaussian processes, Climate emulation

Christian Carmona Perez
Methods for Bayesian modeling under mispecification
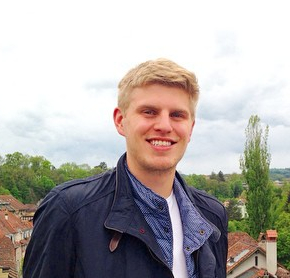
Anthony Caterini
High-Dimensional Statistics, Monte Carlo Methods, Variational Inference
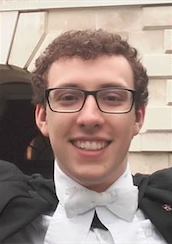
Sam Davenport
Gaussian Processes, fMRI data, Resampling methods, Random Field Theory
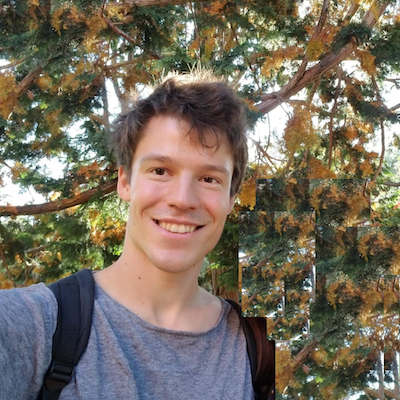
Emilien Dupont
Deep Learning, Generative Models
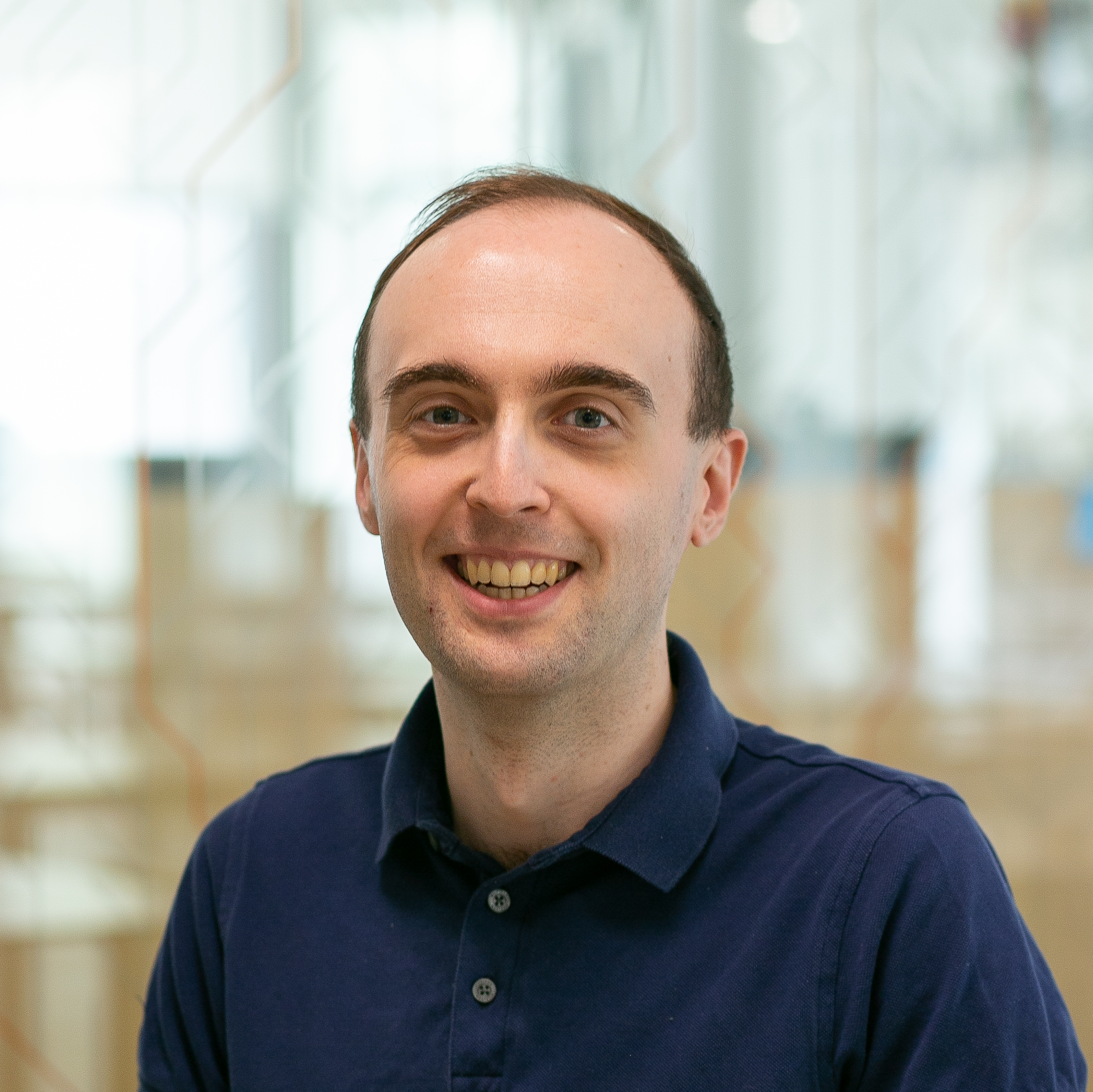
Fabian Falck
Probabilistic Deep Learning, Deep Generative Models, Causality, Applications in Health
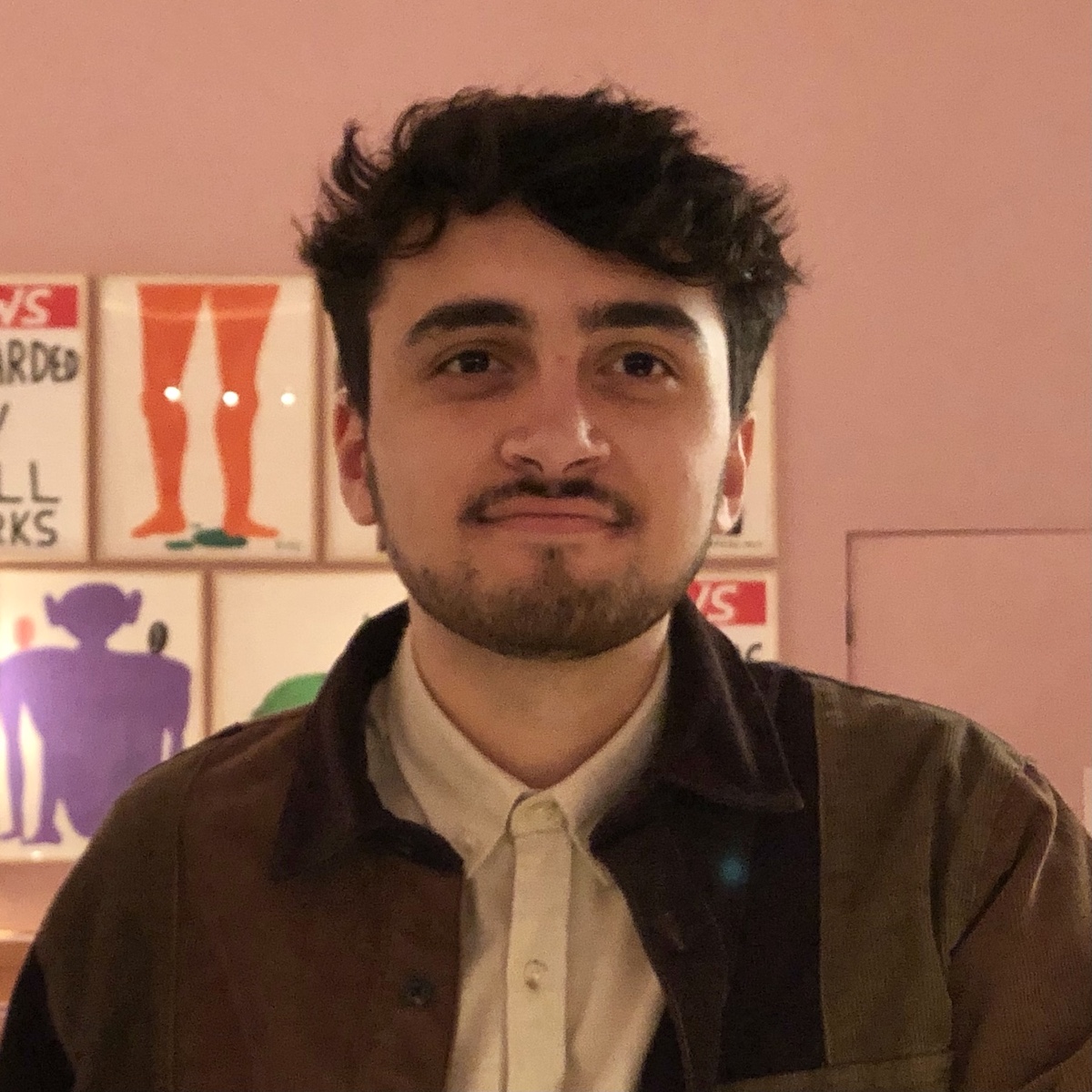
Tyler Farghly
Learning theory, Optimisation, Monte Carlo methods
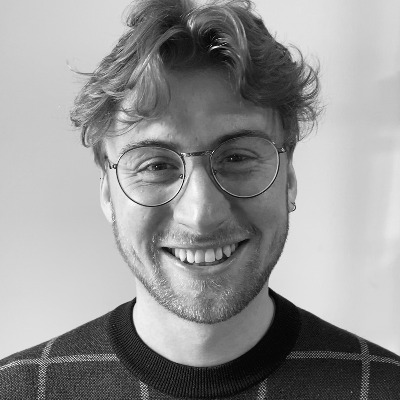
Jake Fawkes
Causal Inference, Machine Learning, Fairness
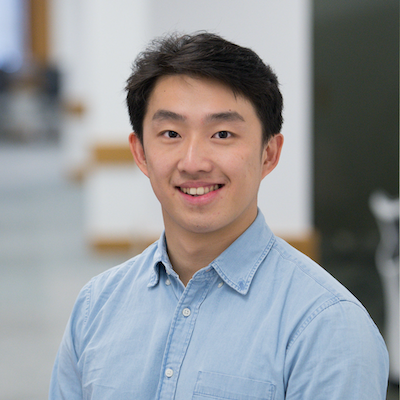
Edwin Fong
Bayesian inference under model misspecification, Bayesian nonparametrics
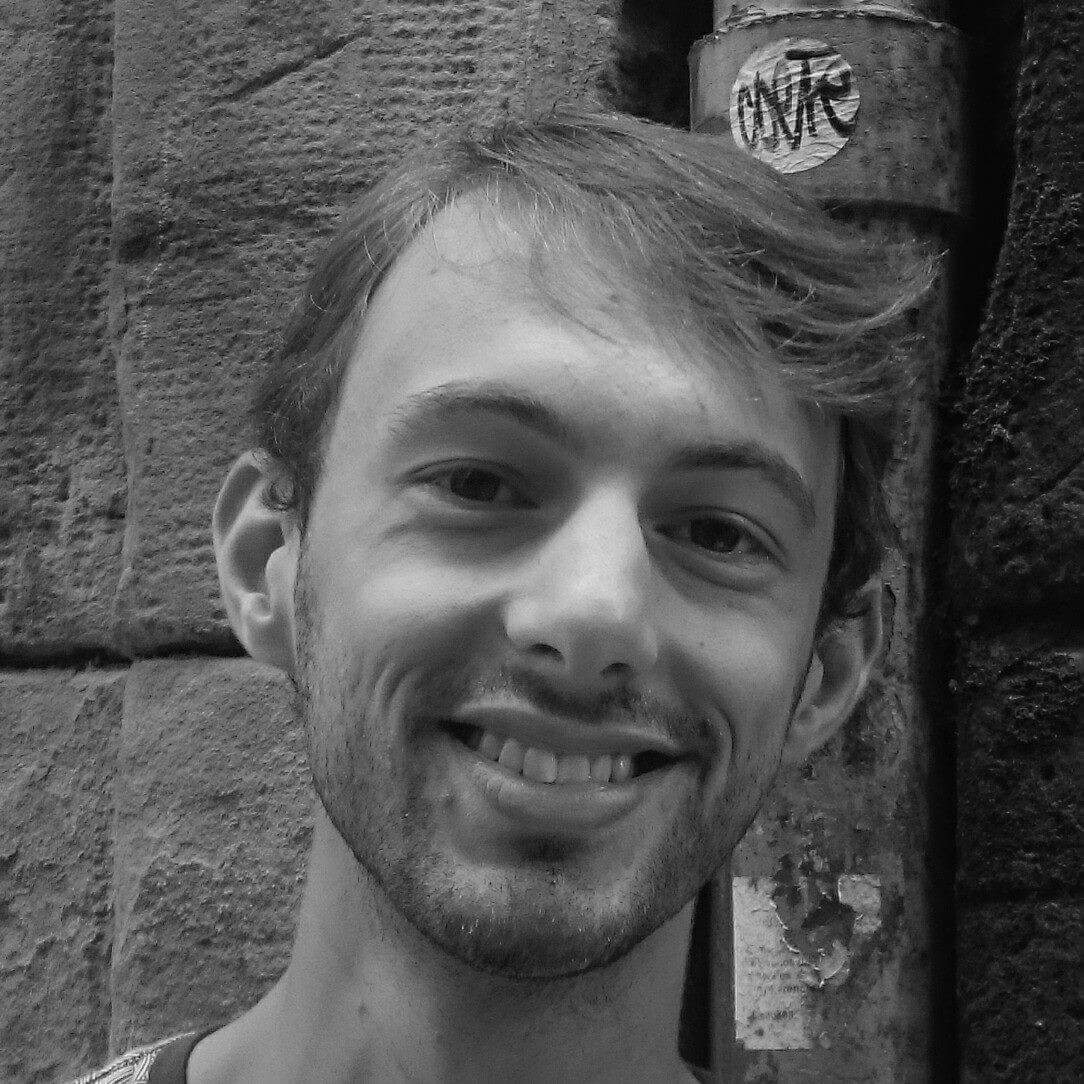
Adam Foster
Probabilistic machine learning, deep learning, unsupervised representation learning, optimal experimental design, probabilistic programming
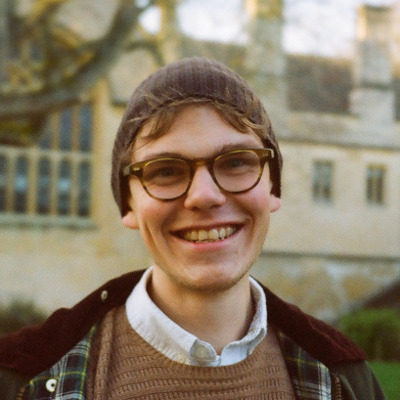
Adam Goliński
Probabilistic Inference, Probablistic Programming
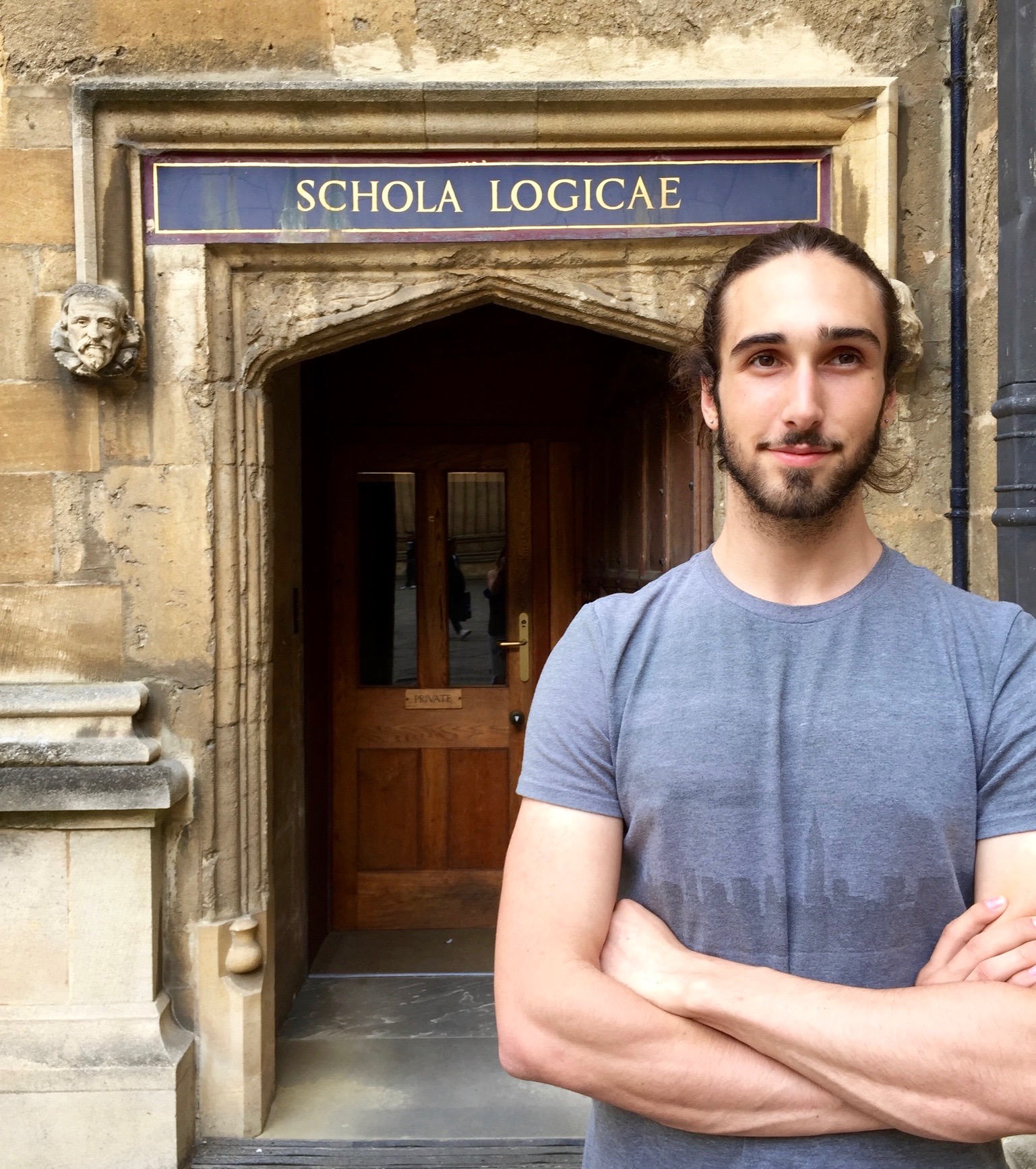
Aidan N. Gomez
Neural networks and deep learning
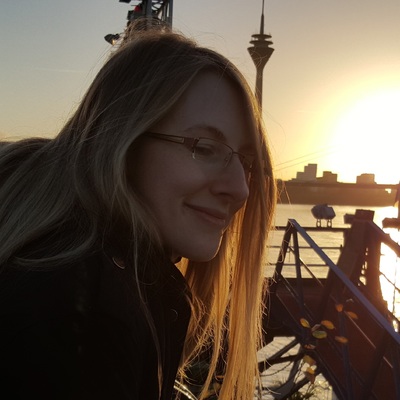
Frauke Harms
combinatorics, computational complexity, Bayesian nonparametrics, machine learning, stochastic geometry
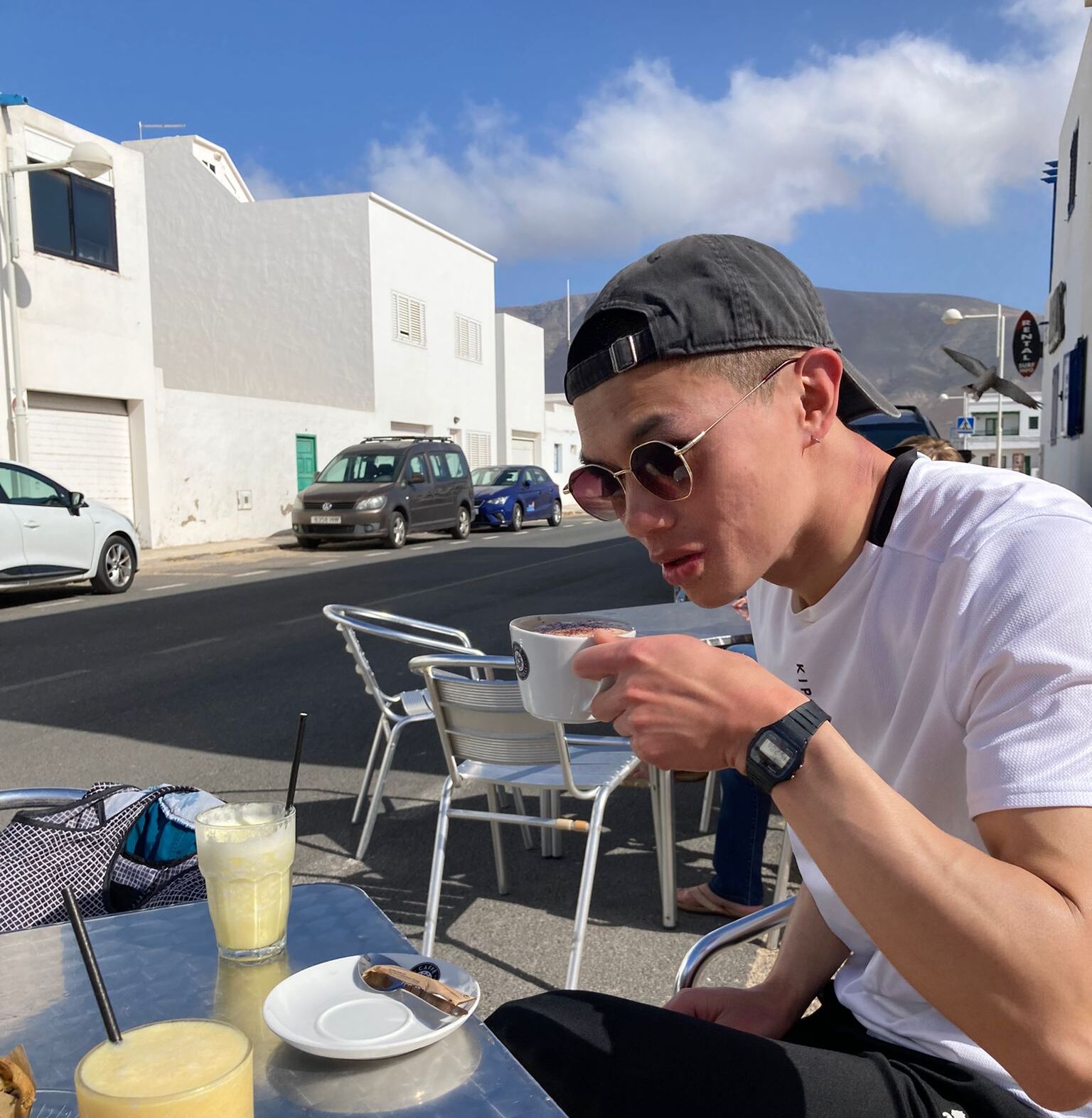
Bobby He
Machine learning, deep learning, uncertainty quantification
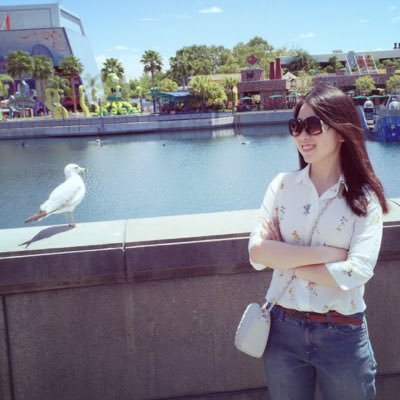
Zhiyuan Hu
Single-Cell Analysis, Ovarian Cancer, Genomics
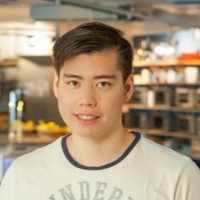
Robert Hu
Machine Learning, Kernel Methods, Causal Inference
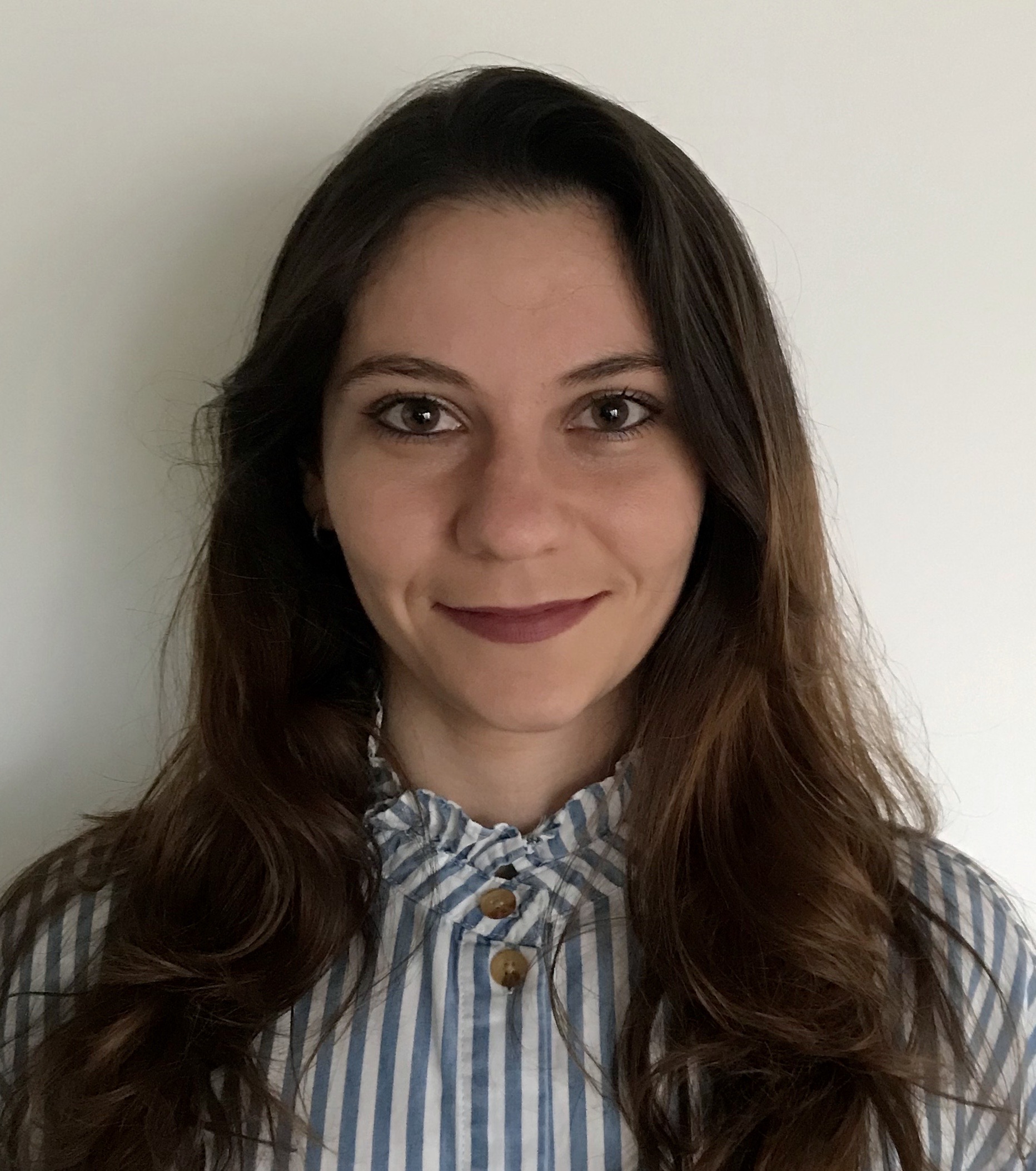
Desi R. Ivanova
Bayesian Inference, Statistical Machine Learning, Optimal Experimental Design
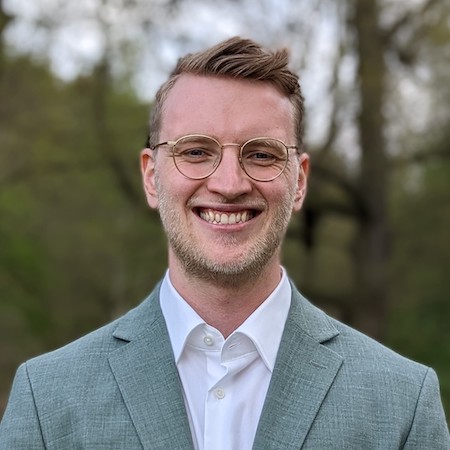
Jannik Kossen
Active Learning, Bayesian Deep Learning, Transformers
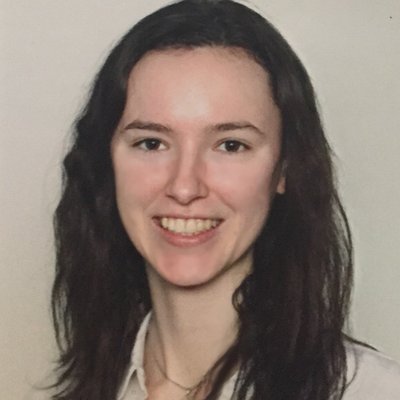
Charline Le Lan
Probabilistic Inference, Deep Learning, Reinforcement Learning
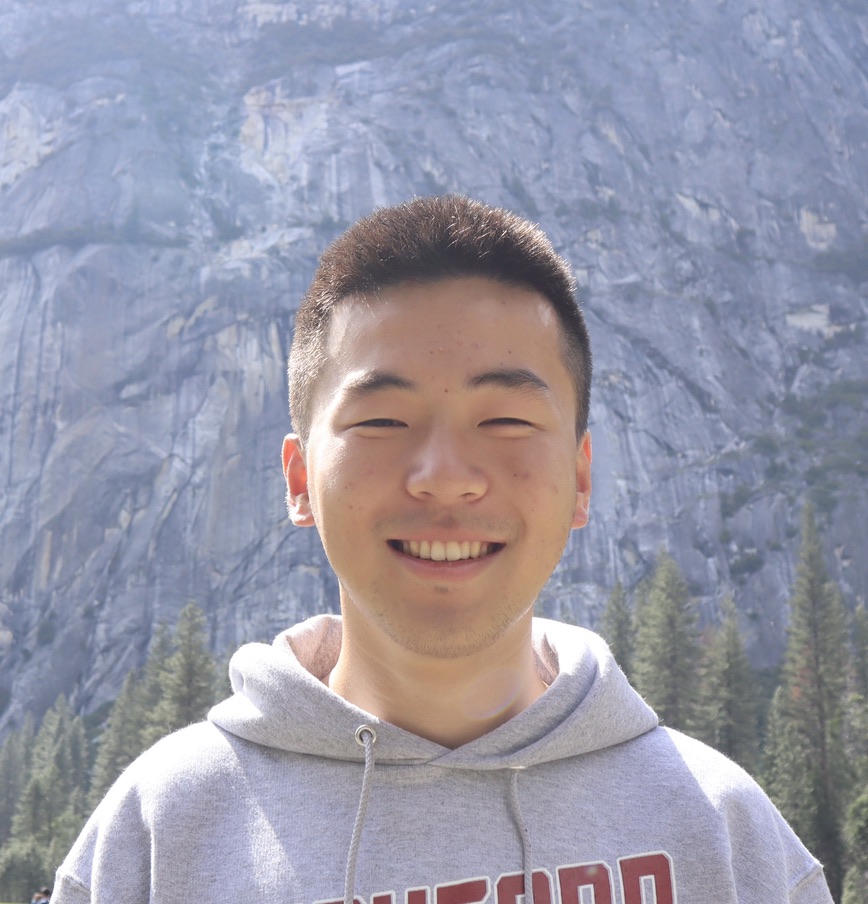
Cong Lu
Deep Reinforcement Learning, Meta-Learning, Bayesian Optimisation
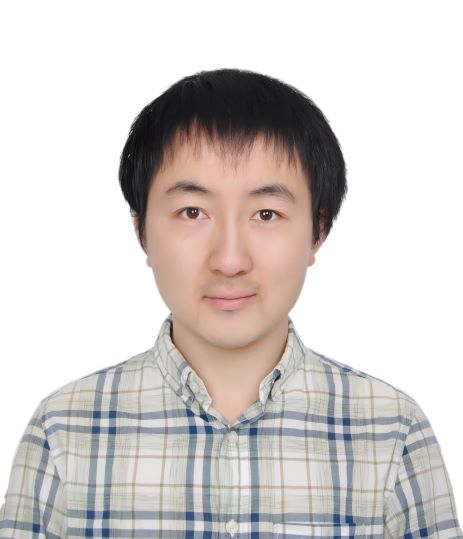
Ning Miao
Deep generative models
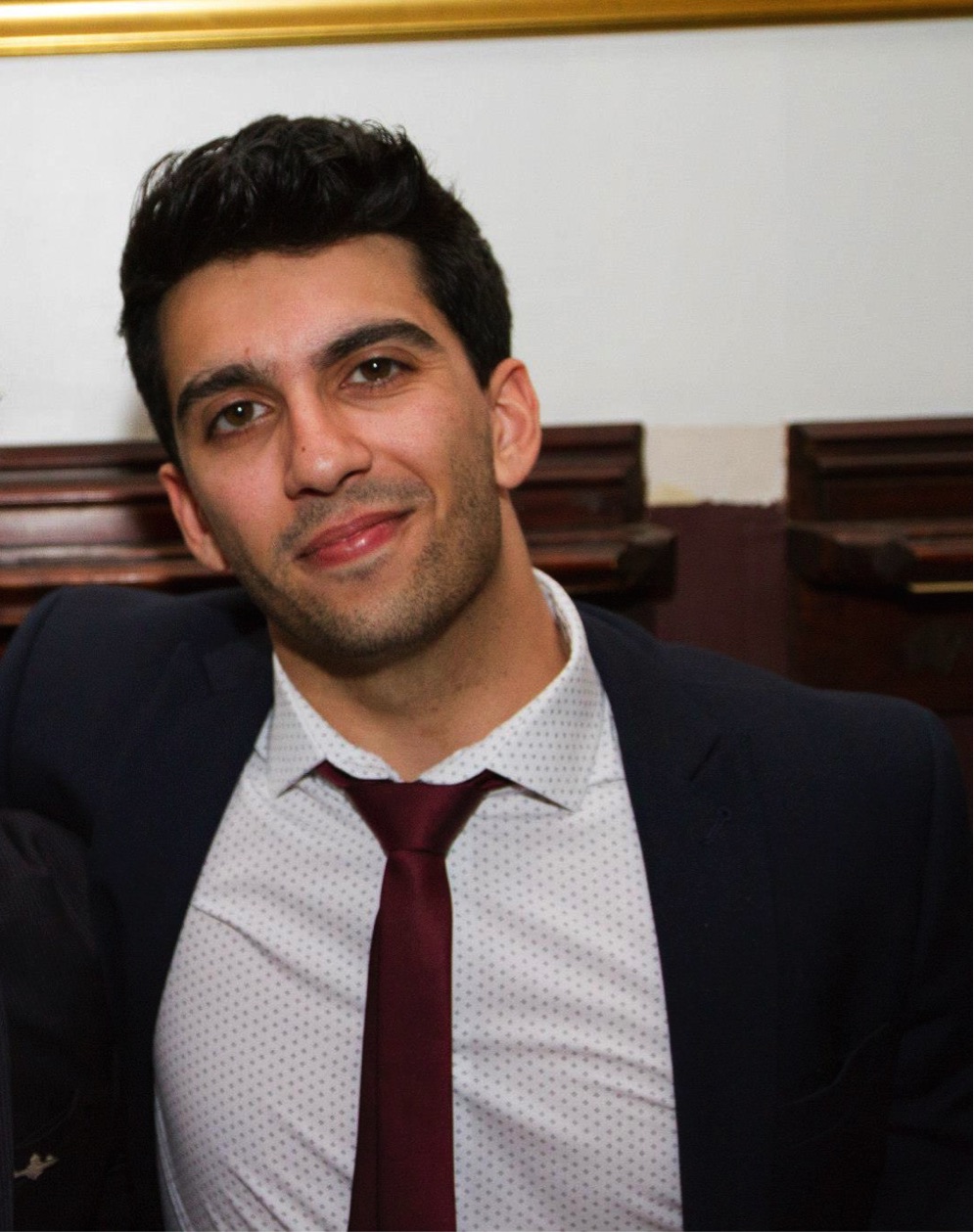
Cian Naik
Bayesian nonparametrics, Statistical Network Analysis
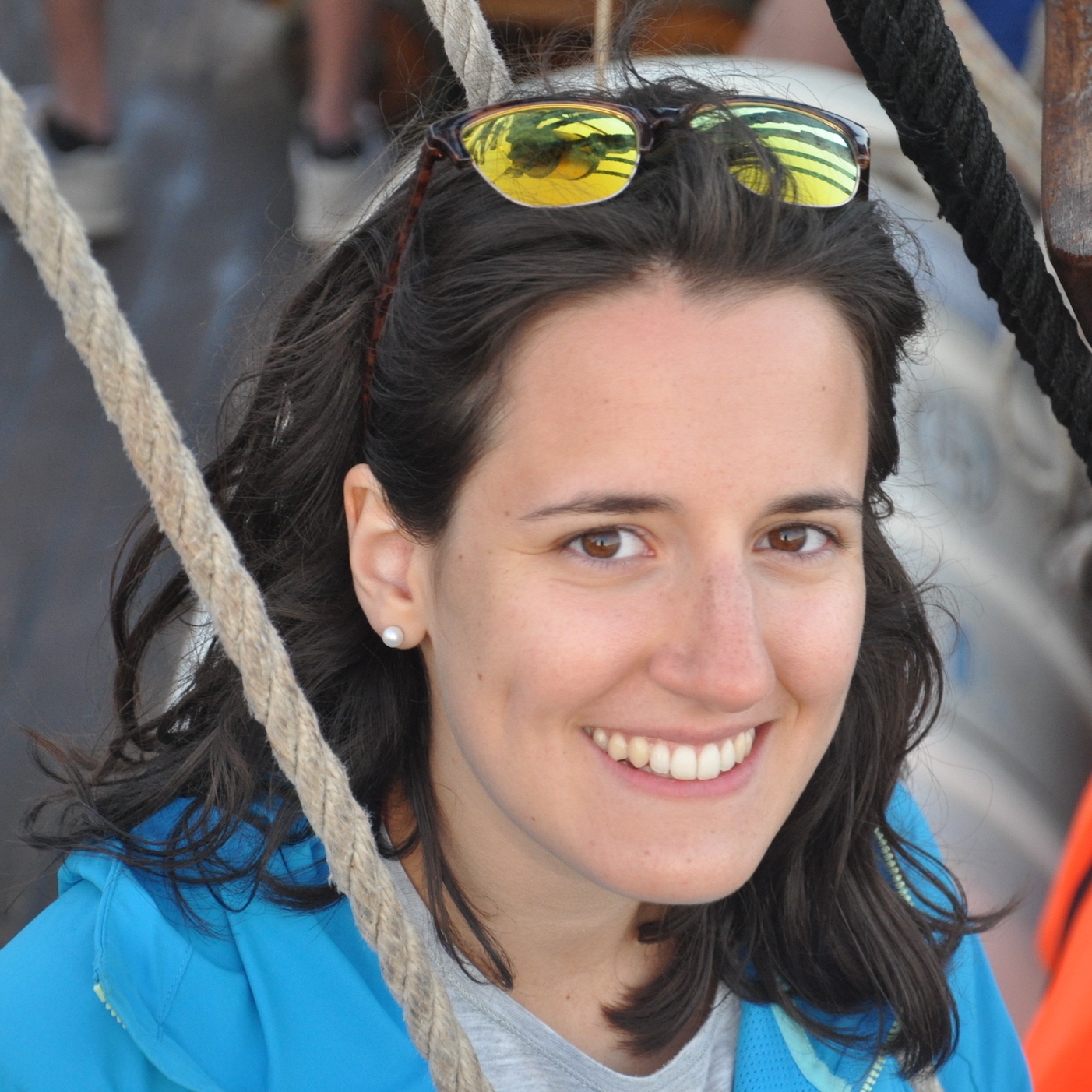
Francesca Panero
Bayesian random graphs, Bayesian nonparametrics, disclosure risk
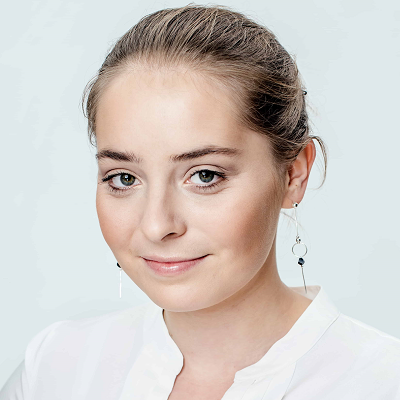
Emilia Pompe
MCMC methods, Bayesian statistics
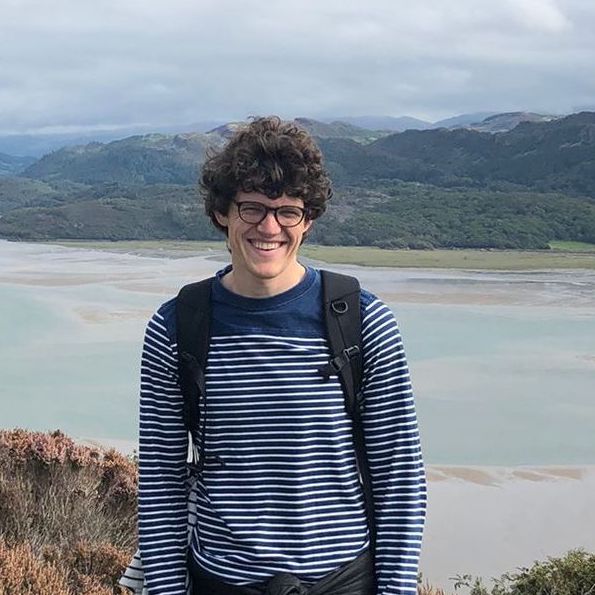
Tim Reichelt
Probabilistic Programming, Probabilistic Inference
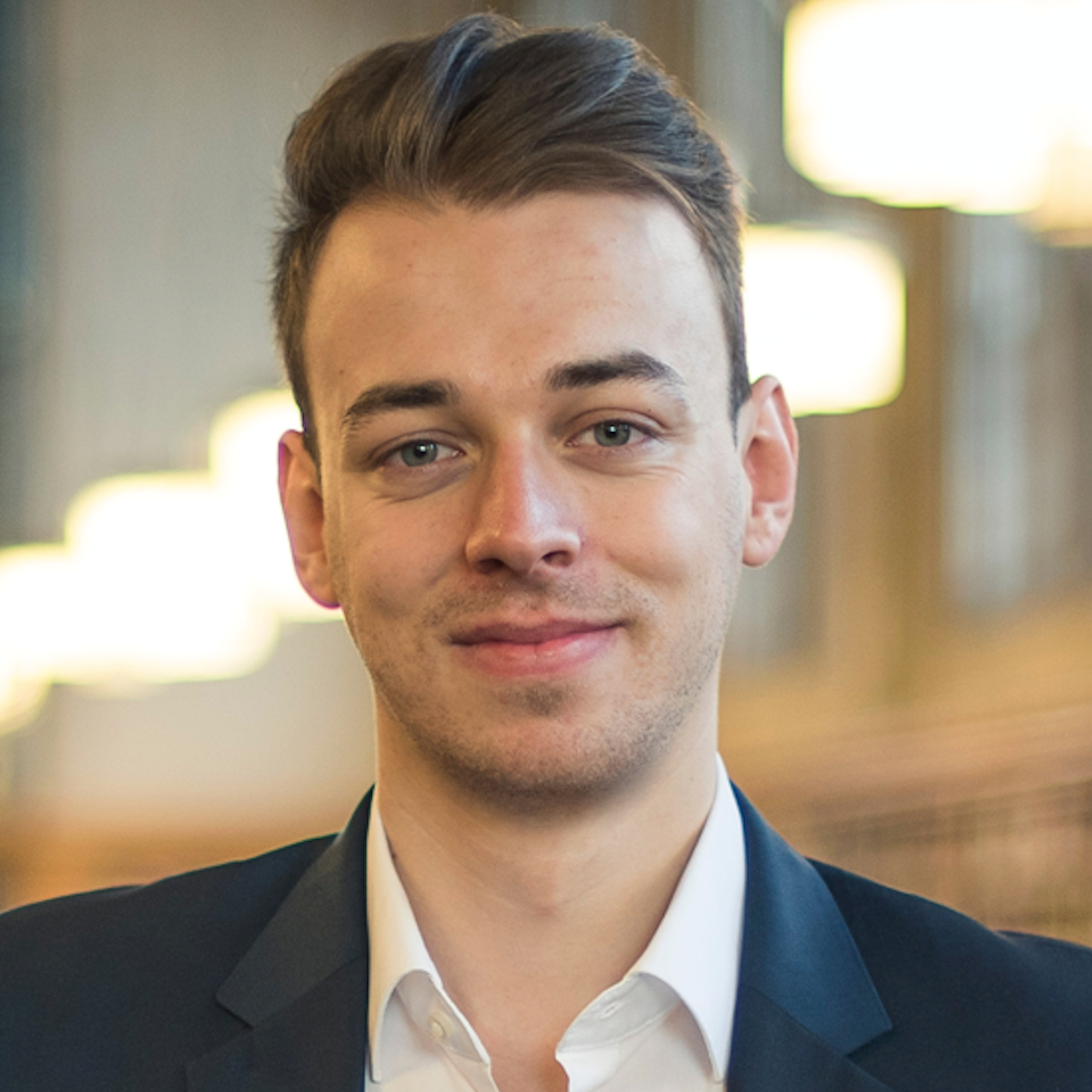
Tim G. J. Rudner
Probabilistic inference, reinforcement learning, Gaussian Processes
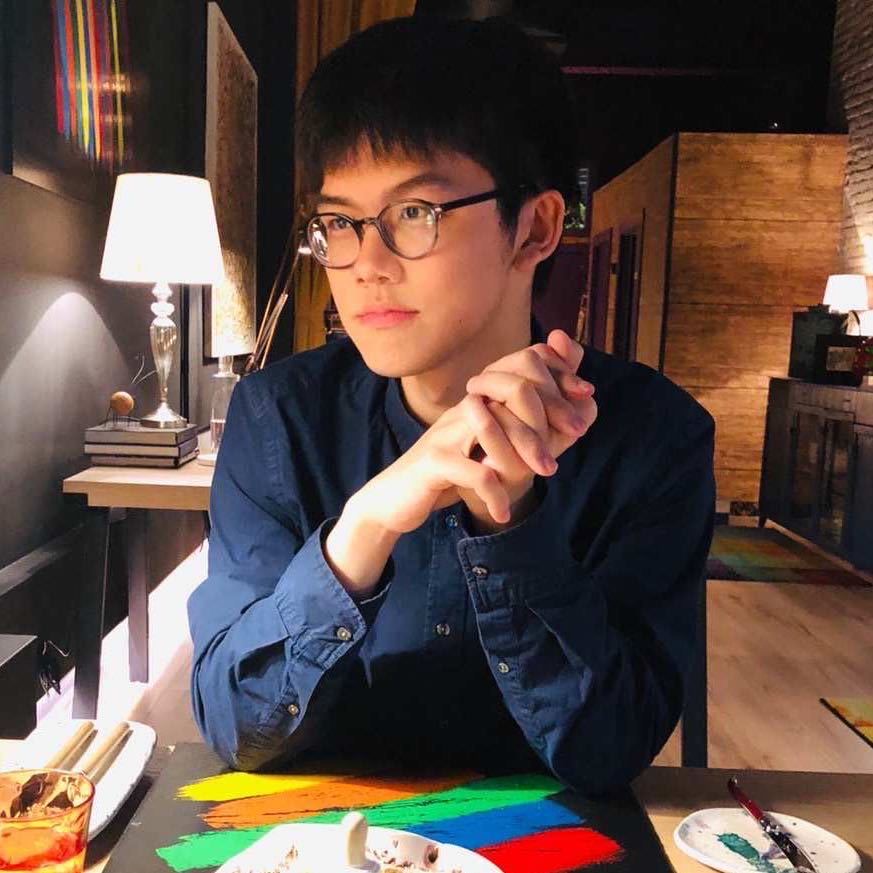
Yuyang Shi
Statistical Machine Learning, Deep Learning, Generative Models
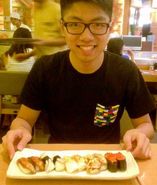
Jean-Francois Ton
Kernel methods, Meta-learning
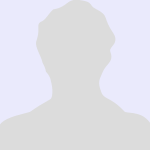
Hanwen Xing
Computational methods, Bayesian inference
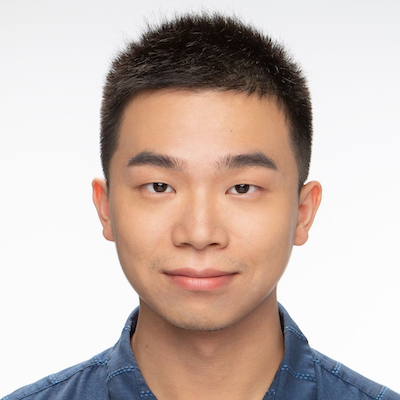
Jin Xu
Meta-learning, equivariance in deep learning
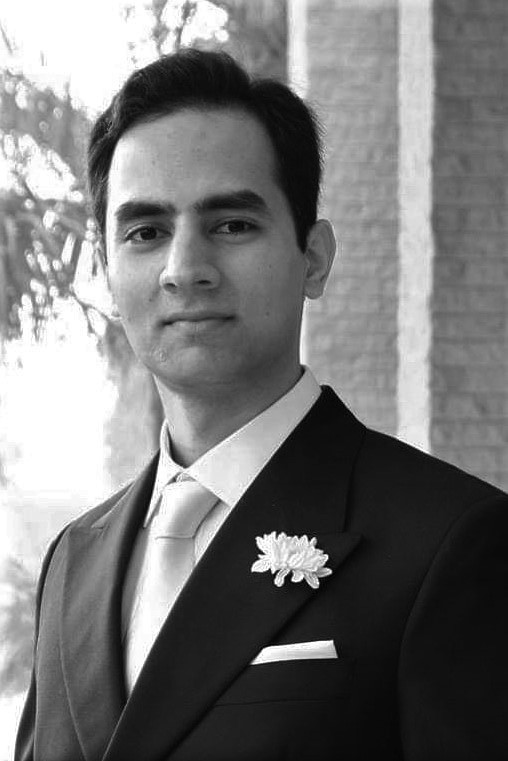
Schyan Zafar
Monte Carlo methods, Multivariate stochastic processes
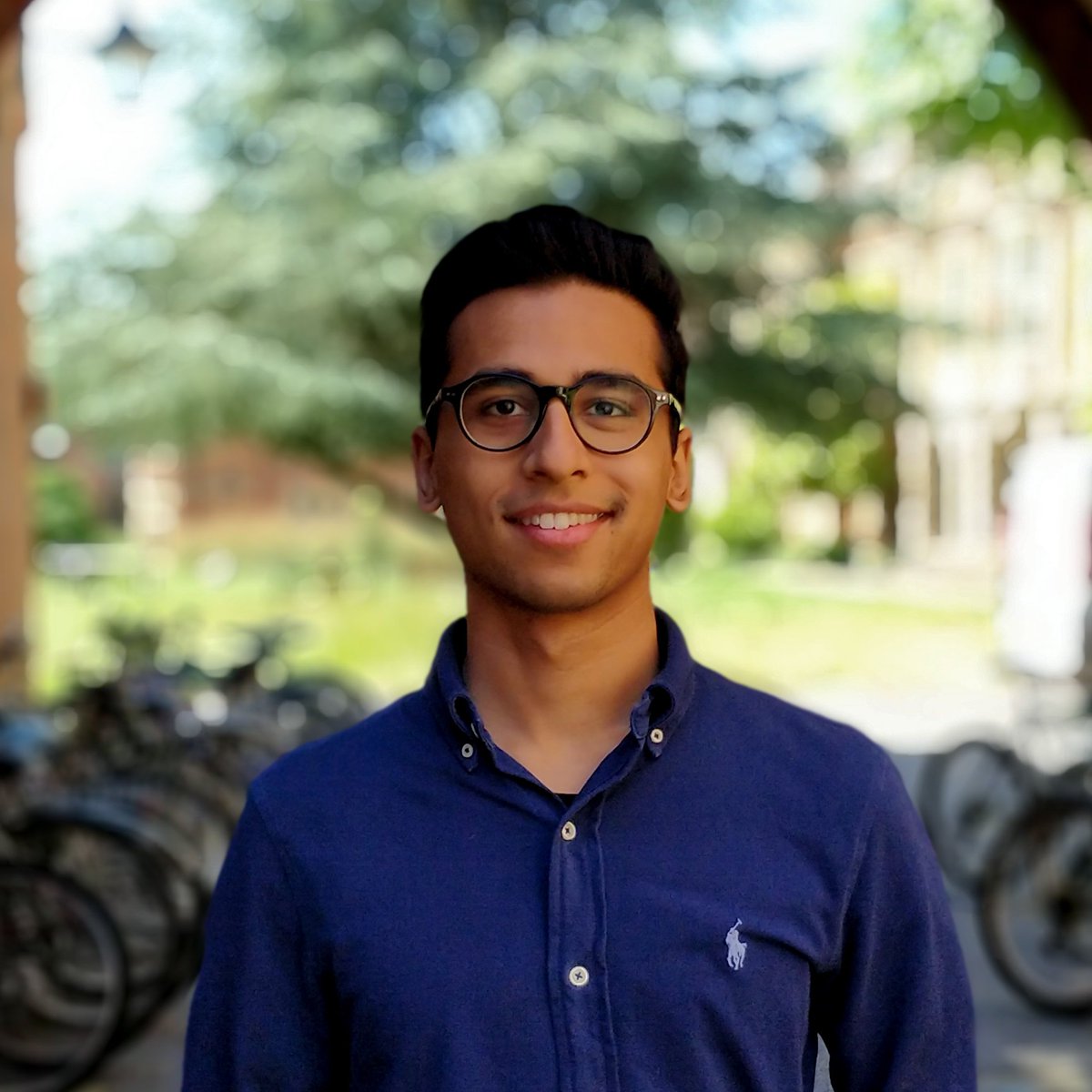
Sheheryar Zaidi
Statistical Machine Learning, Deep Learning
Former Members
- Louis Aslett
- Fadhel Ayed
- Remi Bardenet
- Marco Battiston
- Benjamin Bloem-Reddy
- Levi Boyles
- Ryan Christ
- Giuseppe Di Benedetto
- Lloyd Elliott
- Seth Flaxman
- Bradley Gram-Hansen
- Leonard Hasenclever
- Pierre Jacob
- Yunlong Jiao
- Hyunjik Kim
- Adam R. Kosiorek
- Ho Chung Leon Law
- Juho Lee
- Zhu Li
- Thibaut Lienart
- Xiaoyu Lu
- Simon Lyddon
- Chris J. Maddison
- Kaspar Märtens
- Xenia Miscouridou
- Jovana Mitrovic
- Konstantina Palla
- Valerio Perrone
- Dominic Richards
- David Rindt
- Jennifer Rogers
- Andrew Roth
- Patrick Rubin-Delanchy
- Tammo Rukat
- Sebastian Schmon
- Joost van Amersfoort
- Stefan Webb
- Matthew Willetts
- Chieh-Hsi (Jessie) Wu
- Qinyi Zhang
- Yuan Zhou